Voxweave vs Typeset
When comparing Voxweave vs Typeset, which AI Summarizer tool shines brighter? We look at pricing, alternatives, upvotes, features, reviews, and more.
In a comparison between Voxweave and Typeset, which one comes out on top?
When we put Voxweave and Typeset side by side, both being AI-powered summarizer tools, The upvote count favors Typeset, making it the clear winner. Typeset has received 25 upvotes from aitools.fyi users, while Voxweave has received 6 upvotes.
Feeling rebellious? Cast your vote and shake things up!
Voxweave
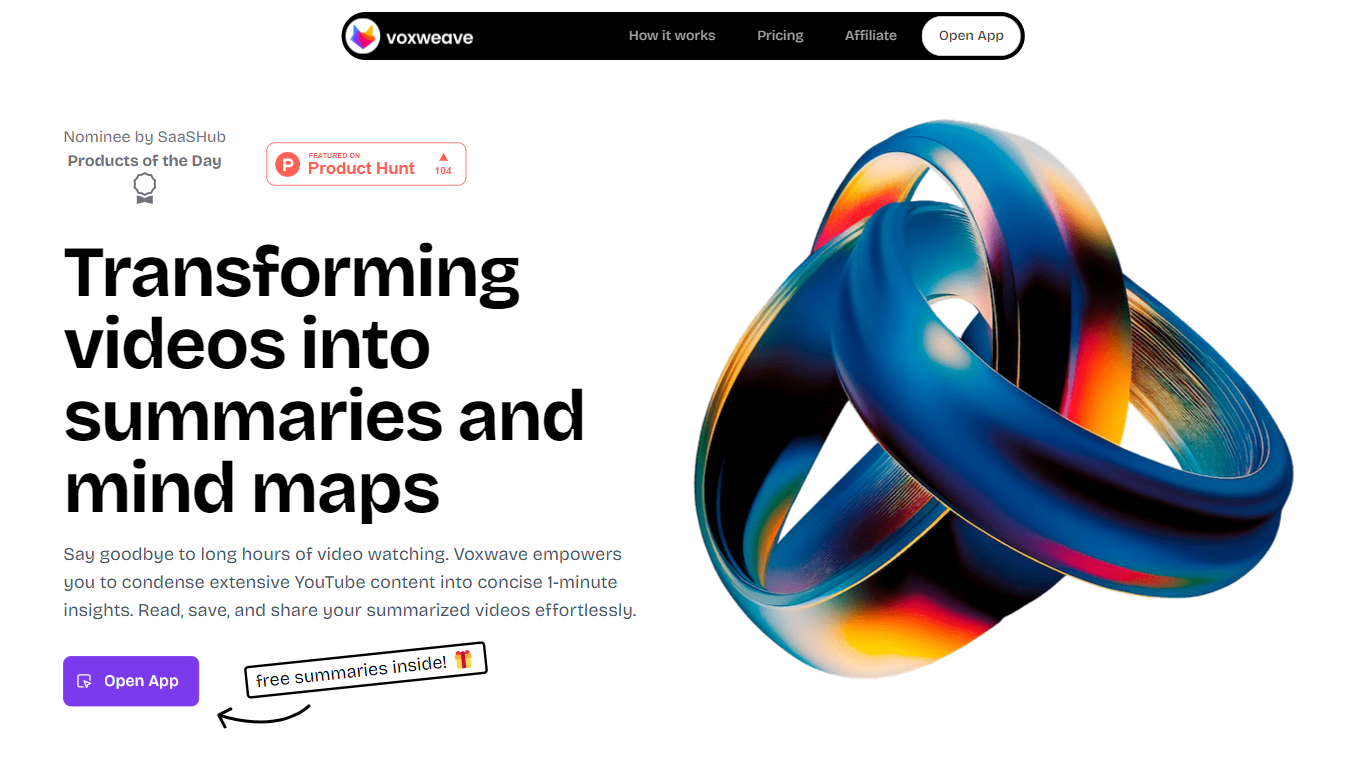
What is Voxweave?
Voxweave offers an AI-powered video summarization tool that simplifies the process of converting YouTube videos into concise text summaries and mind maps. It provides a user-friendly interface that makes it easy to transcribe and summarize video content, enabling users to read at their own pace and absorb information more efficiently. The platform supports a wide array of languages, offering subtitles and automatic translation to English. Voxweave's service is suited for both casual users and professionals, with different subscription plans tailored to individual needs. With its time-saving features and commitment to improving precision, Voxweave is positioned as a valuable tool for content creation, learning, and accessibility enhancement.
Typeset
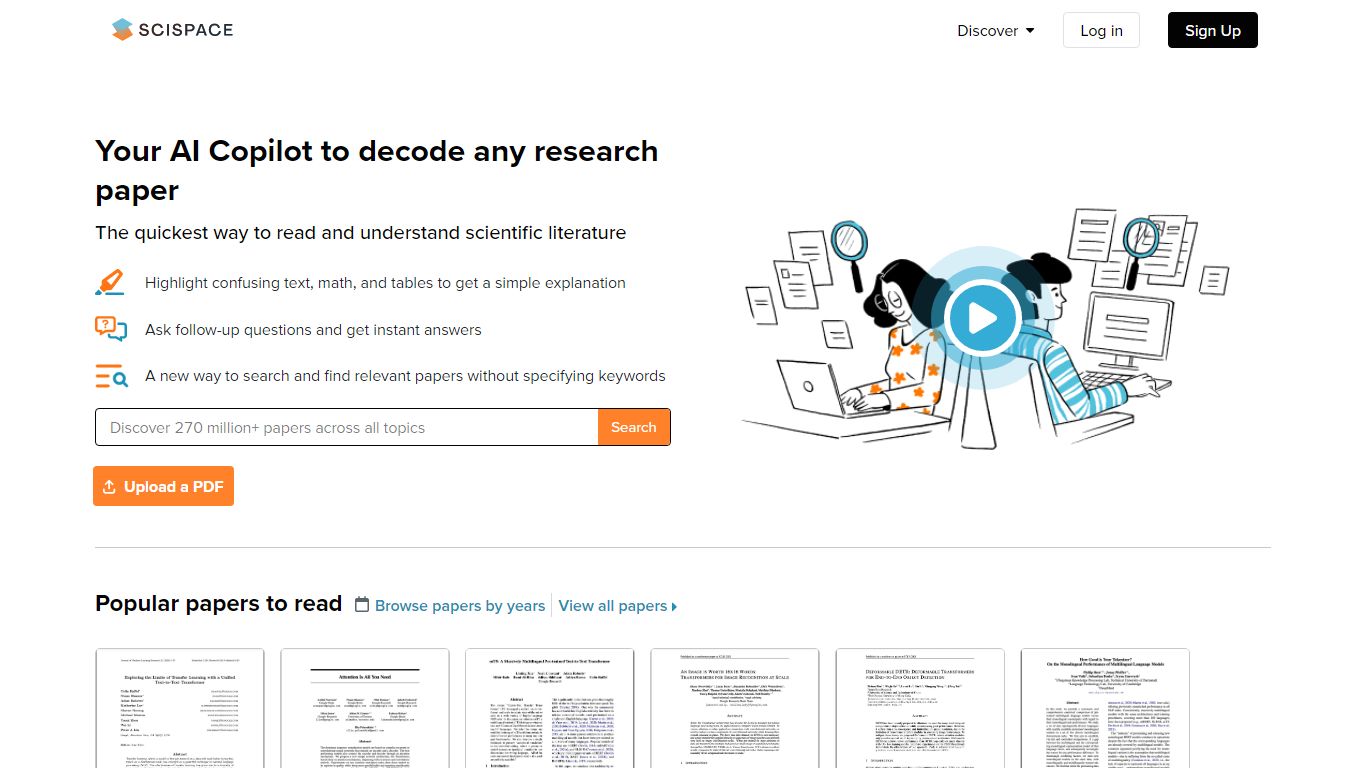
What is Typeset?
Your platform to explore and explain papers. Search for 270M+ papers, understand them in simple language, and find connected papers, authors, topics.
Voxweave Upvotes
Typeset Upvotes
Voxweave Top Features
Simple Video to Text Summarization: Turn lengthy YouTube content into concise, insightful summaries quickly and easily.
Multilingual Support: Supports numerous languages and offers subtitles and automatic translations to English.
Mind Map Generation: Create visual representations of summaries to help understand and remember information better.
Effortless Transcription Process: Paste a YouTube link, press "Summarize", and get high-quality transcriptions and summaries with a few clicks.
Subscription-Based Plans: Offers starter, individual, and business plans to fit various user needs, each with a set number of transcription and summary units.
Typeset Top Features
No top features listedVoxweave Category
- Summarizer
Typeset Category
- Summarizer
Voxweave Pricing Type
- Freemium
Typeset Pricing Type
- Free