Slacksift vs Typeset
Compare Slacksift vs Typeset and see which AI Summarizer tool is better when we compare features, reviews, pricing, alternatives, upvotes, etc.
Slacksift

What is Slacksift ?
Slacksift offers a single-command solution to turn the chaos of Slack threads into structured, accessible conversations. Dealing with a multitude of messages can be overwhelming and time-consuming, but Slacksift addresses this issue by summarizing the key points of any Slack thread, enabling users to catch up and respond quicker.
The app is easy to set up in three simple steps: install, set up with @slacksift setup, and then summarize threads when needed. Its straightforward pricing ensures you pay only for what you use, at a cost of €0.45 per 500 tokens, with no subscription fees or hidden costs. With benefits like unlimited usage and a 48-hour support response time, Slacksift is committed to improving your workflow efficiency.
Typeset
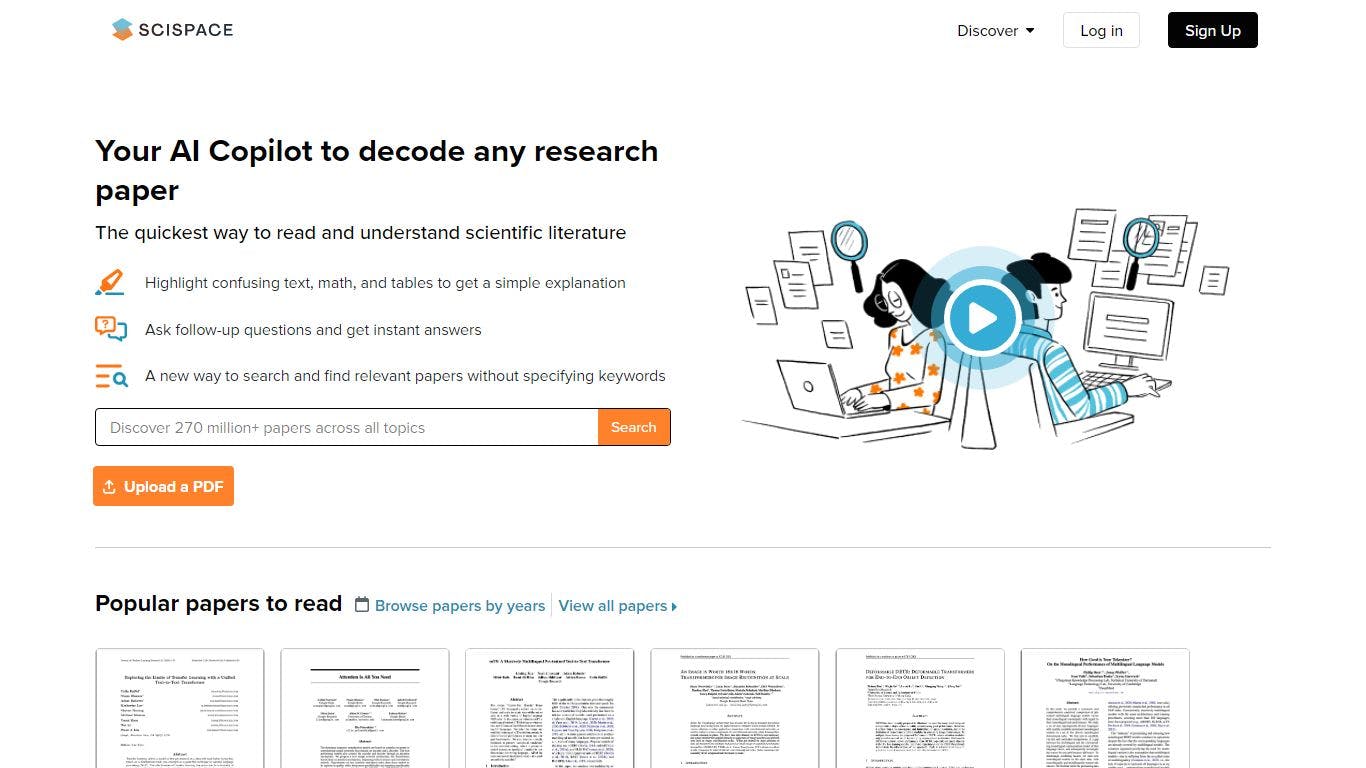
What is Typeset?
Your platform to explore and explain papers. Search for 270M+ papers, understand them in simple language, and find connected papers, authors, topics.
Slacksift Upvotes
Typeset Upvotes
Slacksift Top Features
Efficient Summarization: Quickly understand the key points of Slack threads with a simple @slacksift summarise command.
Easy Installation: Get started with Slacksift within seconds by following an intuitive, three-step setup process.
Usage-Based Pricing: Pay only €0.45 per 500 tokens with no hidden fees or subscriptions.
Fast Catch-Up: Save time by easily catching up with long conversations without reading every single message.
Better Workflow: Streamline Slack interactions and respond faster, enhancing overall productivity.
Typeset Top Features
No top features listedSlacksift Category
- Summarizer
Typeset Category
- Summarizer
Slacksift Pricing Type
- Freemium
Typeset Pricing Type
- Free
Slacksift Technologies Used
Typeset Technologies Used
Slacksift Tags
Typeset Tags
Slacksift Average Rating
No rating availableTypeset Average Rating
Slacksift Reviews
No reviews availableTypeset Reviews
Which one is better? Slacksift or Typeset?
When we compare Slacksift with Typeset, which are both AI-powered summarizer tools, The upvote count favors Typeset, making it the clear winner. Typeset has attracted 24 upvotes from aitools.fyi users, and Slacksift has attracted 6 upvotes.
Not your cup of tea? Upvote your preferred tool and stir things up!