RWKV-LM vs ggml.ai
Dive into the comparison of RWKV-LM vs ggml.ai and discover which AI Large Language Model (LLM) tool stands out. We examine alternatives, upvotes, features, reviews, pricing, and beyond.
In a comparison between RWKV-LM and ggml.ai, which one comes out on top?
When we compare RWKV-LM and ggml.ai, two exceptional large language model (llm) tools powered by artificial intelligence, and place them side by side, several key similarities and differences come to light. Both tools have received the same number of upvotes from aitools.fyi users. The power is in your hands! Cast your vote and have a say in deciding the winner.
Not your cup of tea? Upvote your preferred tool and stir things up!
RWKV-LM
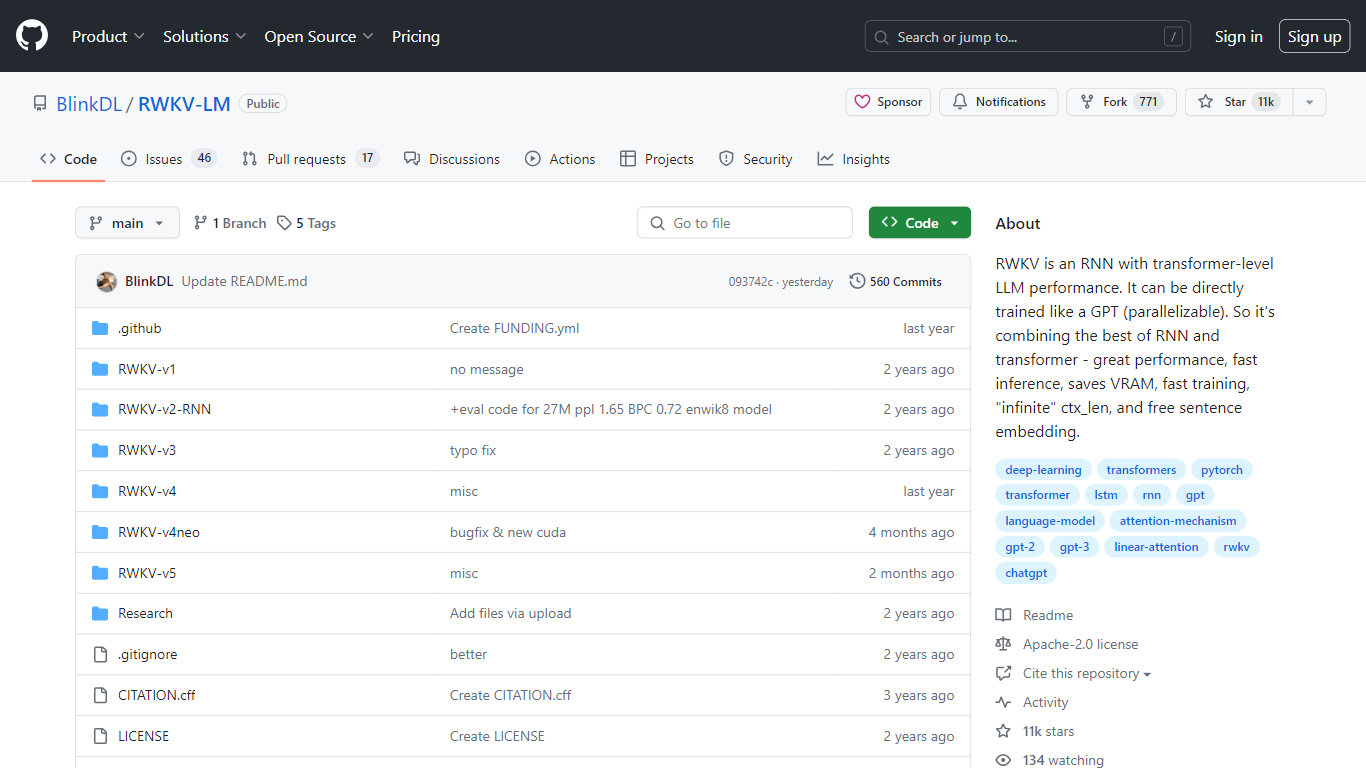
What is RWKV-LM?
RWKV is an innovative RNN-based Language Model that delivers the exceptional performance of transformer-level Large Language Models (LLMs). This remarkable fusion of RNN simplicity with transformer efficiency creates a model that is highly parallelizable, akin to the GPT models. RWKV is not only swift in inference but also demonstrates expedient training speeds while being memory-efficient, thereby conserving valuable VRAM.
It supports an "infinite" context length, allowing it to handle very long sequences of data seamlessly. Furthermore, users benefit from free sentence embedding capabilities, enhancing its utility for a wide array of natural language processing applications. As an Apache-2.0 licensed project, it stands as a public repository on GitHub, inviting collaboration and continued development.
ggml.ai
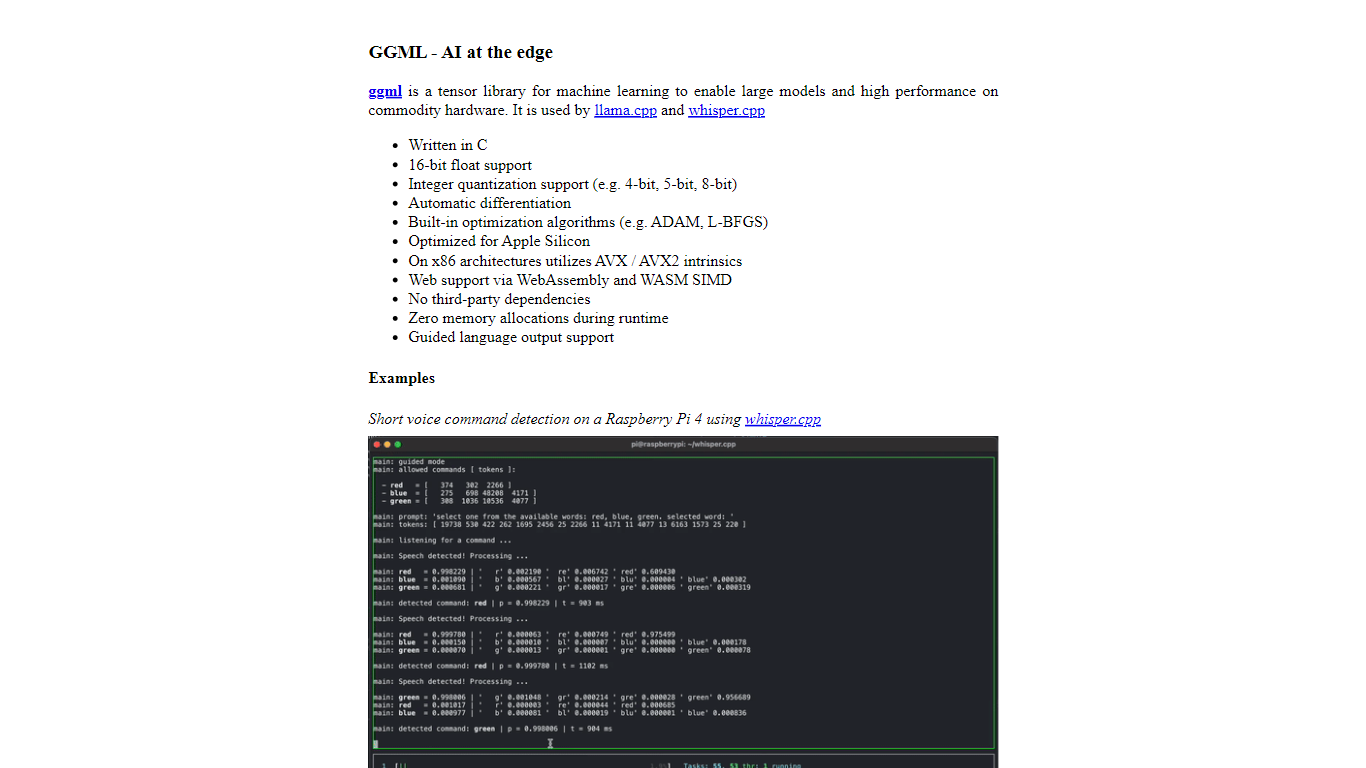
What is ggml.ai?
ggml.ai is at the forefront of AI technology, bringing powerful machine learning capabilities directly to the edge with its innovative tensor library. Built for large model support and high performance on common hardware platforms, ggml.ai enables developers to implement advanced AI algorithms without the need for specialized equipment. The platform, written in the efficient C programming language, offers 16-bit float and integer quantization support, along with automatic differentiation and various built-in optimization algorithms like ADAM and L-BFGS. It boasts optimized performance for Apple Silicon and leverages AVX/AVX2 intrinsics on x86 architectures. Web-based applications can also exploit its capabilities via WebAssembly and WASM SIMD support. With its zero runtime memory allocations and absence of third-party dependencies, ggml.ai presents a minimal and efficient solution for on-device inference.
Projects like whisper.cpp and llama.cpp demonstrate the high-performance inference capabilities of ggml.ai, with whisper.cpp providing speech-to-text solutions and llama.cpp focusing on efficient inference of Meta's LLaMA large language model. Moreover, the company welcomes contributions to its codebase and supports an open-core development model through the MIT license. As ggml.ai continues to expand, it seeks talented full-time developers with a shared vision for on-device inference to join their team.
Designed to push the envelope of AI at the edge, ggml.ai is a testament to the spirit of play and innovation in the AI community.
RWKV-LM Upvotes
ggml.ai Upvotes
RWKV-LM Top Features
Great Performance: Delivers transformer-level LLM performance in a more compact RNN architecture.
Fast Inference: Engineered for quick responses, making it suitable for real-time applications.
VRAM Savings: Optimized to utilize less VRAM without compromising on efficiency.
Fast Training: Able to be trained rapidly, reducing the time needed to develop robust models.
Infinite Context Length: Accommodates extremely long sequences, offering flexibility in processing large amounts of data.
ggml.ai Top Features
Written in C: Ensures high performance and compatibility across a range of platforms.
Optimization for Apple Silicon: Delivers efficient processing and lower latency on Apple devices.
Support for WebAssembly and WASM SIMD: Facilitates web applications to utilize machine learning capabilities.
No Third-Party Dependencies: Makes for an uncluttered codebase and convenient deployment.
Guided Language Output Support: Enhances human-computer interaction with more intuitive AI-generated responses.
RWKV-LM Category
- Large Language Model (LLM)
ggml.ai Category
- Large Language Model (LLM)
RWKV-LM Pricing Type
- Free
ggml.ai Pricing Type
- Freemium