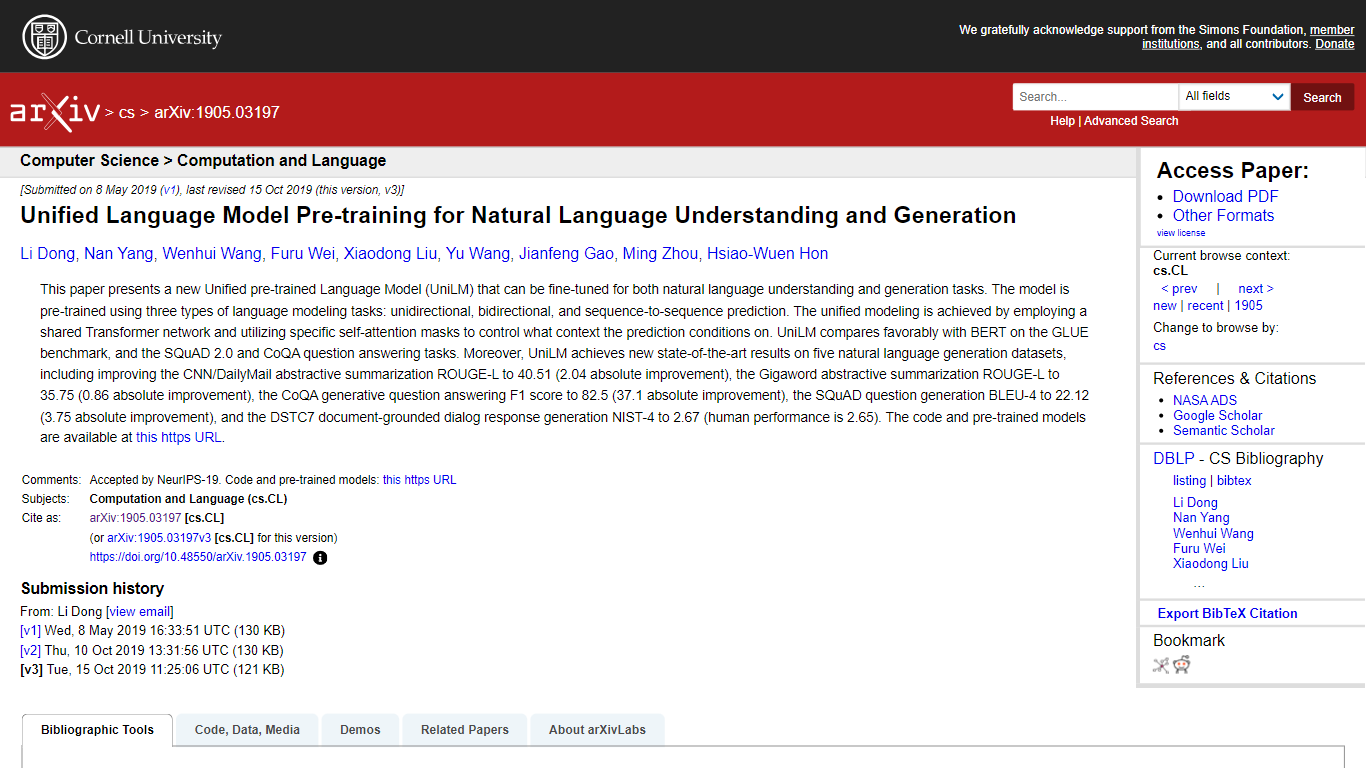
Last updated 02-10-2024
Category:
Reviews:
Join thousands of AI enthusiasts in the World of AI!
UniLM
This paper introduces UniLM, a Unified pre-trained Language Model, that serves as a new benchmark for Natural Language Understanding (NLU) and Natural Language Generation (NLG) tasks. It is unique in its use of a shared Transformer network that is pre-trained on unidirectional, bidirectional, and sequence-to-sequence tasks, employing special self-attention masks for contextual prediction control. UniLM outperforms BERT in the GLUE benchmark and excels in SQuAD 2.0 and CoQA question answering, setting new records in five NLG datasets, including notable improvements in CNN/DailyMail and Gigaword summarization tasks. The models and code shared by the authors aid the research community in further advancements.
Comprehensive Pre-training: UniLM is pre-trained on unidirectional, bidirectional, and sequence-to-sequence language modeling tasks.
Dual-purpose Design: Optimized for both natural language understanding and generation, making it a versatile tool in NLP.
Superior Self-Attention Control: Unique self-attention masks in the shared Transformer network allow context-specific predictions.
Benchmark Excellence: Achieves new state-of-the-art results on several benchmarks, surpassing previous models like BERT.
Open Source Contribution: Authors provide access to pre-trained models and code for community use and improvement.
1) What is UniLM?
UniLM stands for Unified pre-trained Language Model and is designed for both natural language understanding and generation tasks.
2) How is UniLM pre-trained?
The model is pre-trained using unidirectional, bidirectional, and sequence-to-sequence language modeling tasks.
3) Does UniLM perform better than BERT?
Yes, UniLM outperforms BERT on the GLUE benchmark as well as SQuAD 2.0 and CoQA question answering tasks.
4) What accomplishments has UniLM achieved?
New state-of-the-art results were achieved on five NLG datasets, including improvements in CNN/DailyMail and Gigaword summarization tasks.
5) Where can I find the code and pre-trained models for UniLM?
You can access the code and pre-trained models at the GitHub repository provided by the authors.