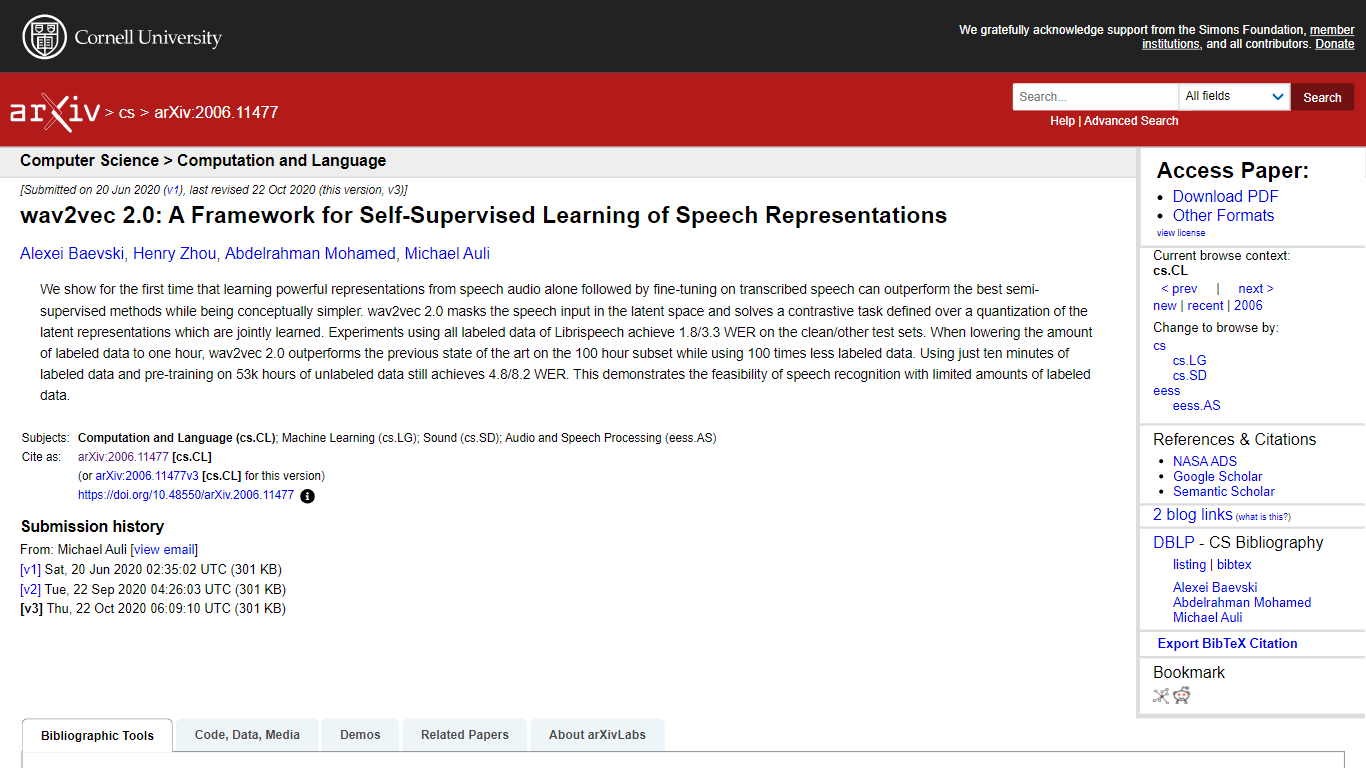
Last updated 02-11-2024
Category:
Reviews:
Join thousands of AI enthusiasts in the World of AI!
wav2vec 2.0
Discover the innovative research presented in the paper titled "wav2vec 2.0: A Framework for Self-Supervised Learning of Speech Representations," which showcases a groundbreaking approach in speech processing technology. This paper, authored by Alexei Baevski, Henry Zhou, Abdelrahman Mohamed, and Michael Auli, introduces the wav2vec 2.0 framework, designed to learn representations from speech audio alone. By fine-tuning on transcribed speech, it outperforms many semi-supervised methods, proving to be a simpler yet potent solution. Key highlights include the ability to mask speech input in the latent space and address a contrastive task over quantized latent representations. The study demonstrates impressive results in speech recognition with a minimal amount of labeled data, changing the landscape for developing efficient and effective speech recognition systems.
Self-Supervised Framework: Introduces wav2vec 2.0 as a self-supervised learning framework for speech processing.
Superior Performance: Demonstrates that the framework can outperform semi-supervised methods while maintaining conceptual simplicity.
Contrastive Task Approach: Employs a novel contrastive task within the latent space to enhance learning.
Minimal Labeled Data: Achieves significant speech recognition results with extremely limited amounts of labeled data.
Extensive Experiments: Shares experimental results utilizing the Librispeech dataset to showcase the framework's effectiveness.
1) What is wav2vec 2.0?
Wav2vec 2.0 is a framework for self-supervised learning of speech representations that masks speech input in the latent space and solves a contrastive task over a quantization of these representations.
2) Who authored the wav2vec 2.0 paper?
Alexei Baevski, Henry Zhou, Abdelrahman Mohamed, and Michael Auli are the authors of the wav2vec 2.0 paper.
3) Can wav2vec 2.0 outperform semi-supervised methods?
Yes, the wav2vec 2.0 framework can outperform semi-supervised methods by learning from speech audio and fine-tuning on transcribed speech.
4) What is a contrastive task in the context of wav2vec 2.0?
A contrastive task in the context of wav2vec 2.0 refers to a method where the framework learns to distinguish between the correct latent representations of input speech and distractor samples.
5) What WER results were achieved using wav2vec 2.0 in experiments?
Experiments with wav2vec 2.0 achieved a 1.8/3.3 WER on Librispeech's clean/other test sets with full labeled data and 4.8/8.2 WER with just ten minutes of labeled data after pre-training on 53k hours of unlabeled data.